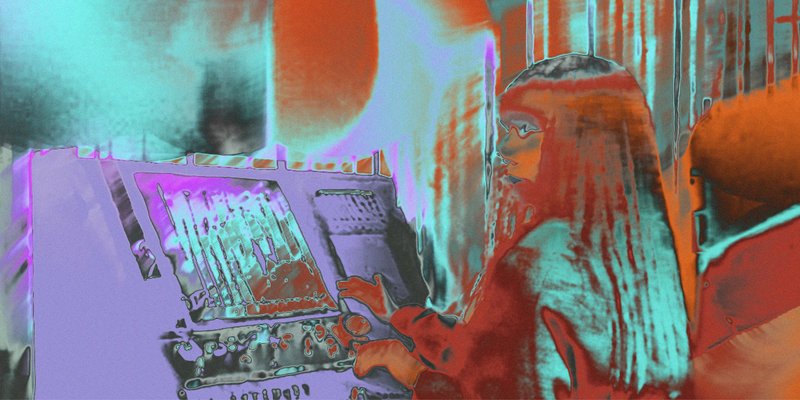
This is Part 1 of two-part aricle. Read Part 2 here.
The word âAIâ provokes mixed emotions. It can inspire excitement and hope for the future - or a shiver of dread at whatâs to come. In the last few years, AI has gone from a distant promise to a daily reality. Many of us use ChatGPT to write emails and Midjourney to generate images. Each week, it seems, a new AI technology promises to change another aspect of our lives.
Music is no different. AI technology is already being applied to audio, performing tasks from stem separation to vocal deepfakes, and offering new spins on classic production tools and music-making interfaces. One day soon, AI might even make music all by itself.
The arrival of AI technologies has sparked heated debates in music communities. Ideas around creativity, ownership, and authenticity are being reexamined. Some welcome what they see as exciting new tools, while others say the technology is overrated and wonât change all that much. Still others are scared, fearing the loss of the music-making practices and cultures they love.
In this two-part article, we will take a deep dive into AI music-making to try to unpick this complex and fast-moving topic. Weâll survey existing AI music-making tools, exploring the creative possibilities they open up and the philosophical questions they pose. And we will try to look ahead, examining how AI tools might change music-making in the future.
The deeper you go into the topic, the stronger those mixed emotions become. The future might be bright, but itâs a little scary too.
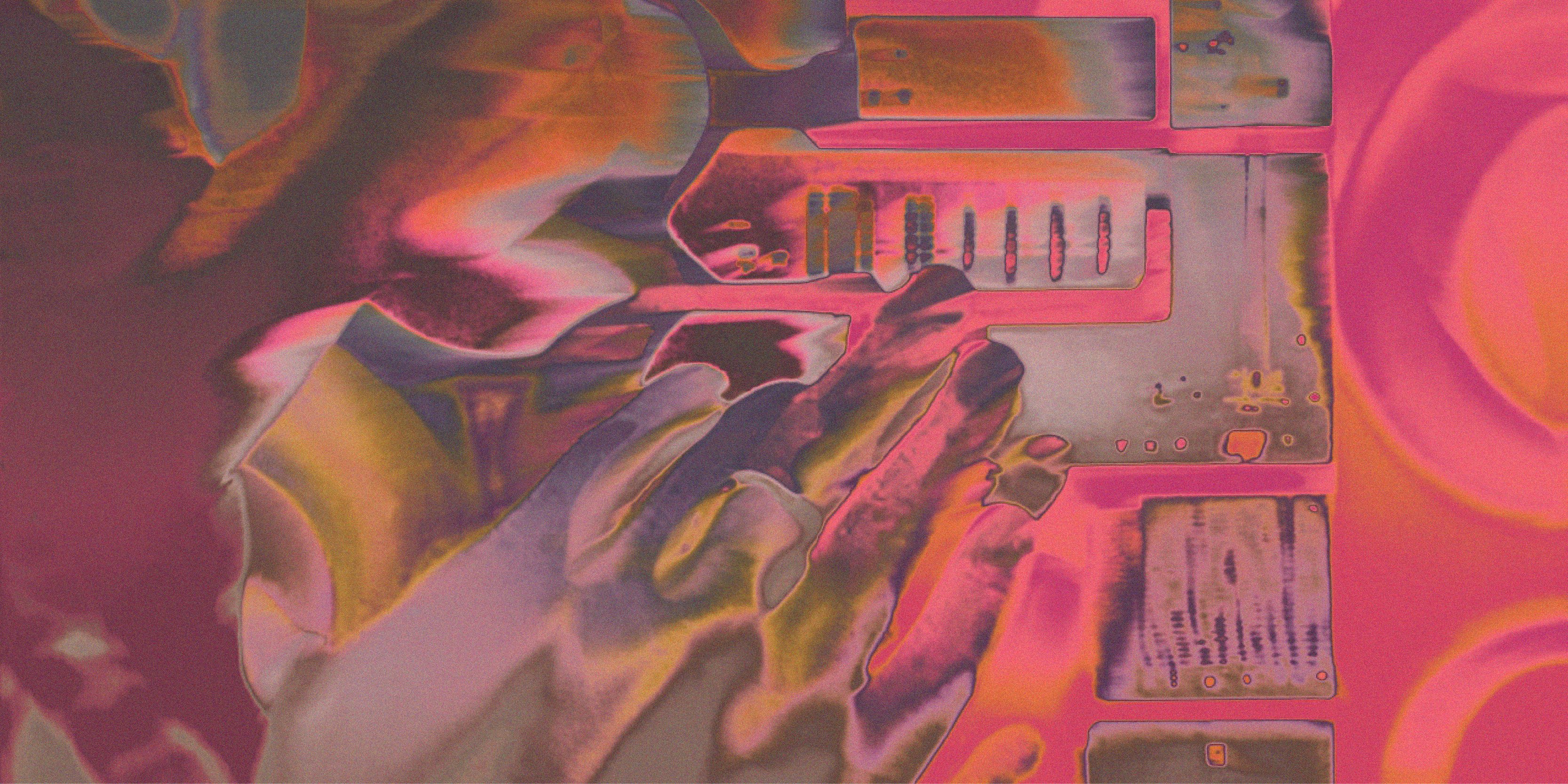
Defining terms
Before we go any further, we should get some terms straight.
First of all, what is AI? The answer isnât as simple as you might think. Coined in the 1950s, the term has since been applied to a range of different technologies. In its broadest sense, AI refers to many forms of computer program that seem to possess human-like intelligence, or that can do tasks that we thought required human intelligence.Â
The AI boom of the last few years rests on a specific technology called machine learning. Rather than needing to be taught entirely by human hand, a machine learning system is able to improve itself using the data itâs fed. But machine learning has been around for decades. Whatâs new now is a specific kind of machine learning called deep learning.Â
Deep learning systems are made up of neural networks: a set of algorithms roughly configured like a human brain, that can interpret incoming data and recognize patterns. The âdeepâ part tells us that there are multiple layers to these networks, allowing the system to interpret data in more sophisticated ways. This makes a deep learning system very skilled at making sense of unstructured data. In other words, you can throw random pictures or text at it and it will do a good job of spotting the patterns.Â
But deep learning systems arenât âintelligentâ in the way often depicted in dystopian sci-fi movies about runaway AIs. They donât possess a âconsciousnessâ as we would understand it - they are just very good at spotting the patterns in data. For this reason, some argue that the term âAIâ is a misnomer.Â
The sophistication of deep learning makes it processor-hungry, hence the technology only becoming widely accessible in the last few years. But deep learning technology has been present in our lives for longer, and in more ways, than you might think. Deep learning is used in online language translators, credit card fraud detection, and even the recommendation algorithms in music streaming services.Â
These established uses of deep learning AI mostly sit under the hood of products and services. Recently, AI has stepped into the limelight. Tools such as Dall-E and ChatGPT donât just sift incoming data to help humans recognise the patterns. They produce an output that attempts to guess what the data will do next. This is called generative AI.Â
Where other forms of deep learning chug along in the background of daily life, generative AI draws attention to itself. By presenting us with images, text, or other forms of media, it invites us into a dialogue with the machine. It mirrors human creativity back at us, and makes the potentials - and challenges - of AI technology more starkly clear.
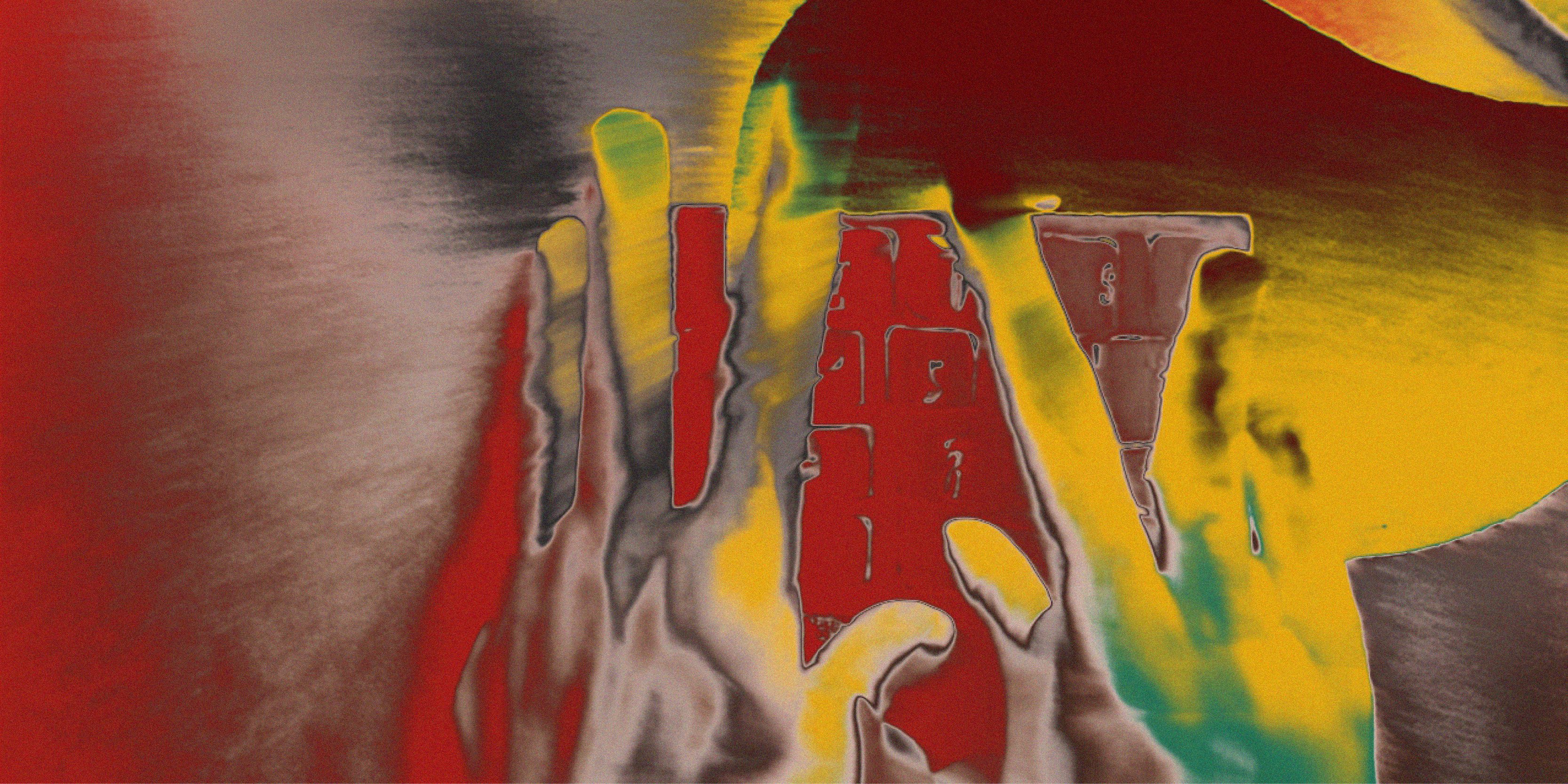
No ChatGPT for music?
Deep learning technology can be applied to digital audio just as it can to images, text, and other forms of data. The implications of this are wide-ranging, and weâll explore them in depth in these articles. But AI audio is lagging behind some other applications of the technology. There is, as yet, no ChatGPT for music. That is: thereâs no tool trained on massive amounts of audio that can accept text or other kinds of prompts and spit out appropriate, high-quality music. (Although there may be one soon - more on this in Part 2).Â
There are a few possible reasons for this. First of all, audio is a fundamentally different kind of data to image or text, as Christian Steinmetz, an AI audio researcher at Queen Mary University, explains. â[Audio] has relatively high sample rate - at each point in time you get one sample, assuming itâs monophonic audio. But you get 44,000 of those samples per second.â This means that generating a few minutes of audio is the data equivalent of generating an absolutely enormous image.Â
As AI audio researchers and innovators the Dadabots observe, this puts a limit on how fast currently available systems can work. âSome of the best quality methods of generating raw audio can require up to a day to generate a single song.â
Unlike images or text, audio has a time dimension. It matters to us how the last minute of a song relates to the first minute, and this poses specific challenges to AI. Music also seems harder to reliably describe in words, making it resistant to the text-prompt approach that works so well for images. âMusic is one of our most abstract artforms,â say the Dadabots. âThe meaning of timbres, harmonies, rhythms alone are up to the listener's interpretation. It can be very hard to objectively describe a full song in a concise way where others can instantly imagine it.â
Added to this, our auditory perception seems to be unusually finely tuned. âWe may be sensitive to distortions in sound in a different way than our visual system is sensitive,â says Steinmetz. He gives the example of OpenAIâs Jukebox, a generative music model launched in 2020 - the most powerful at the time. It could create âsuper convincing musicâ in the sense that the important elements were there. âBut it sounded really bad from a quality perspective. Itâs almost as if for audio, if everything is not in the exact right place, even an untrained listener is aware that there's something up. But for an image it seems like you can get a lot of the details mostly right, and it's fairly convincing as an image. You don't need to have every pixel exactly right.â
Itâs tempting to conclude that music is simply too hard a nut to crack: too mysterious, too ephemeral an aesthetic experience to be captured by the machines. That would be naive. In fact, efforts to design effective AI music tools have been progressing quickly in recent years.Â
There is a race on to create a âgeneral music modelâ - that is, a generative music AI with a versatility and proficiency equivalent to Stable Diffusion or ChatGPT. We will explore this, and its implications for music-making, in Part 2 of this series.
But there are many potential uses for AI in music beyond this dream of a single totalizing system. From generative MIDI to whacky-sounding synthesis, automated mixing to analog modeling, AI tools have the potential to shake up the music-making process. In Part 1, weâll explore some of whatâs out there now, and get a sense of how these tools might develop in the future. In the process, weâll address what these tools might mean for music-making. Does AI threaten human creativity, or simply augment it? Which aspects of musical creation might change, and which will likely stay the same?
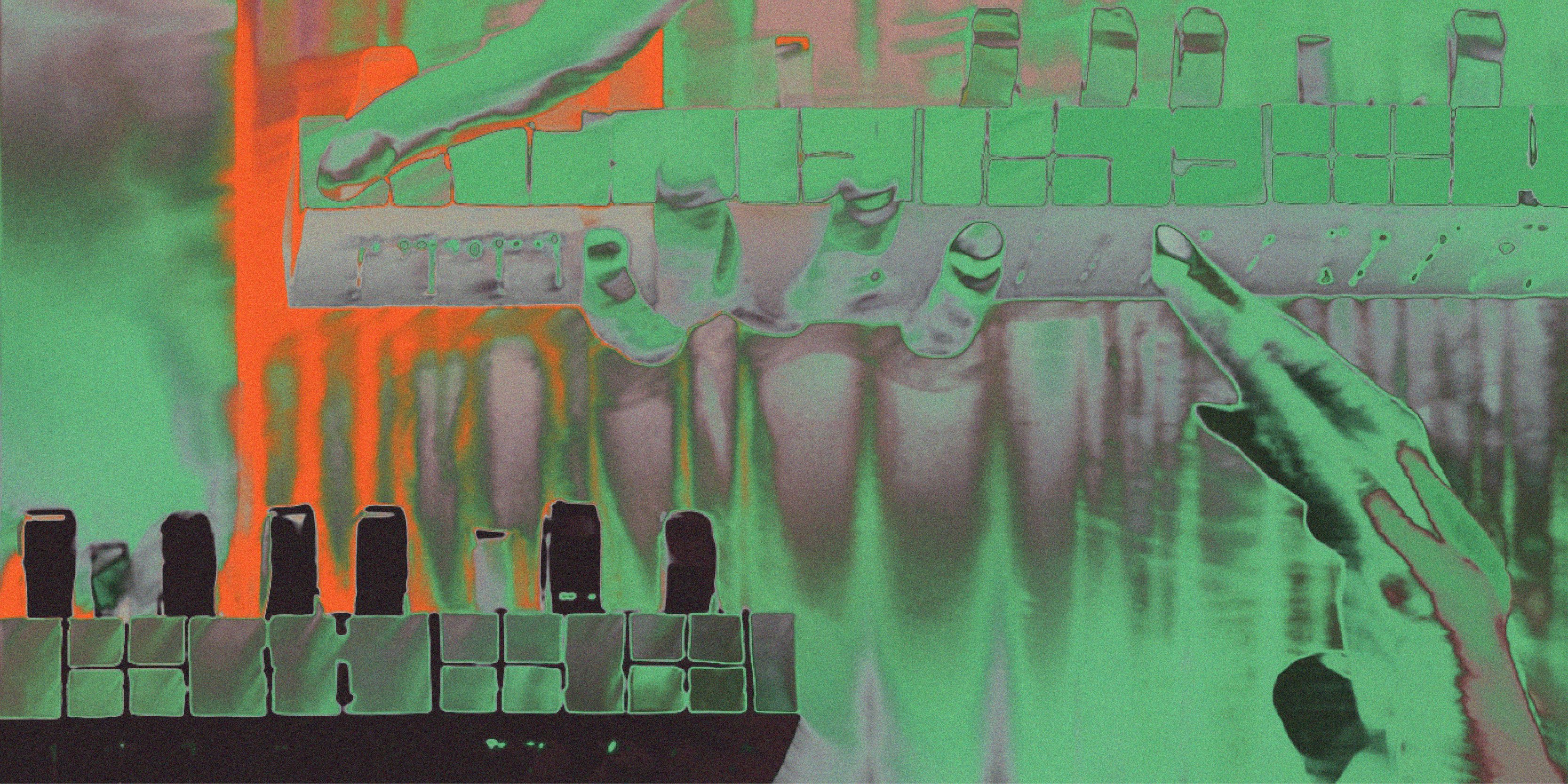
Automating production tasks
At this point you may be confused. If you are a music producer or other audio professional, âAI music production toolsâ might not seem like such a novel idea. In fact, the âAIâ tag has been floating around in the music tech world for years.Â
For example, iZotope have integrated AI into products like their all-in-one mixing tool, Neutron 4. The plug-inâs Mix Assistant listens to your whole mix and analyzes the relationships between the sounds, presenting you with an automated mix that you can tweak to taste.
Companies like Sonible, meanwhile, offer âsmartâ versions of classic plug-in effects such as compression, reverb, and EQ. These plug-ins listen to the incoming audio and adapt to it automatically. The user is then given a simpler set of macro controls for tweaking the settings. pure:comp, for instance, offers just one main âcompressionâ knob that controls parameters such as threshold, ratio, attack, and release simultaneously.Â
Other tools offer to automate parts of the production process that many producers tend to outsource. LANDR will produce an AI-automated master of your track for a fraction of the cost of hiring a professional mastering engineer. You simply upload your premaster to their website, choose between a handful of mastering styles and loudness levels, and download the mastered product.Â
What is the relationship between these tools and the deep learning technologies that are breaking through now? Here we come back to the vagueness of the term âAI.â Deep learning is one kind of AI technology, but itâs not the only one. Before that, we had âexpert systems.âÂ
As Steinmetz explains, this method works âby creating a tree of options.â He describes how an automated mixing tool might work following this method. âIf the genre is jazz, then you go to this part of the tree. If itâs jazz and the instrument is an upright bass, then you go to this part of the tree. If it's an upright bass and there's a lot of energy at 60 hertz, then maybe decrease that. You come up with a rule for every possible scenario. If you can build a complicated enough set of rules, you will end up with a system that appears intelligent.â
"If you're doing a job that could theoretically be automated - meaning that no one cares about the specifics of the artistic outputs, we just need it to fit some mold - then that job is probably going to be automated eventually."
Itâs difficult to say for sure what technology is used in individual products. But itâs likely that AI-based music tech tools that have been around for more than a few years use some variation of this approach. (Of course, deep learning methods may have been integrated into these tools more recently). Â
This approach is effective when executed well, but it has limitations. As Steinmetz explains, such technology requires expert audio engineers to sit down with programmers and write all the rules. And as anyone who has mixed a track will know, itâs never so simple as following the rules. A skilled mix engineer makes countless subtle decisions and imaginative moves. The number of rules youâd need to fully capture this complexity is simply too vast. âThe problem is of scale, basically,â says Steinmetz.
Hereâs where deep learning comes in. Remember: deep learning systems can teach themselves from data. They donât need to be micromanaged by a knowledgeable human. The more relevant data theyâre fed, and the more processor power they have at their disposal, the more proficient they can become at their allotted task.Â
This means that a deep learning model fed on large amounts of music would likely do a better job than an expert systems approach - and might, by some metrics, even surpass a human mix engineer.
This is not yet a reality in the audio domain, but Steinmetz points to image classification as an example of AI tools reaching this level. âThe best model is basically more accurate than a human at classifying the contents of an image, because we've trained it on millions of images - more images than a human would even be able to look at. So that's really powerful.â
This means that AI will probably get very good at various technical tasks that music producers have until now considered an essential part of the job. From micro-chores like setting your compressorâs attack and decay, to diffuse tasks like finalizing your entire mixdown, AI may soon be your very own in-house engineer.Â
How will this change things for music-makers? Steinmetz draws an analogy with the democratization of digital photography through smartphone cameras. Professional photographers who did workaday jobs like documenting events lost out; demand for fine art photographers stayed the same.
âIn mixing or audio engineering, it's a similar thing. If you're doing a job that could theoretically be automated - meaning that no one cares about the specifics of the artistic outputs, we just need it to fit some mold - then that job is probably going to be automated eventually.â But when a creative vision is being realized, the technology wonât be able to replace the decision-maker. Artists will use âthe AI as a tool, but they're still sitting in the pilot's seat. They might let the tool make some decisions, but at the end of the day, they're the executive decision-maker.â
Of course, this wonât be reassuring to those who make their living exercising their hard-won production or engineering skills in more functional ways. We might also wonder whether the next generation of producers could suffer for it. There is a creative aspect to exactly how you compress, EQ, and so on. If technology automates these processes, will producers miss out on opportunities to find creative new solutions to age-old problems - and to make potentially productive mistakes?
On the other hand, by automating these tasks, music-makers will free up time and energy - which they can spend expanding the creative scope of their music in other ways. Many tasks that a current DAW executes in seconds would, in the era of analog studios, have taken huge resources, work hours, and skill. We donât consider the music made on modern DAWs to be creatively impoverished as a result. Instead, the locus of creativity has shifted, as new sounds, techniques, and approaches have become accessible to more and more music-makers.
âIt is true that some aspects of rote musical production are likely to be displaced by tools that might make light work of those tasks,â says Mat Dryhurst, co-founder - alongside his partner, the musician Holly Herndon - of the AI start-up Spawning. âBut that just shifts the baseline for what we consider art to be. Generally speaking artists we cherish are those that deviate from the baseline for one reason or another, and there will be great artists in the AI era just as there have been great artists in any era.â

In the beginning there was MIDI
Making a distinction between functional production tasks and artistry is relatively easy when thinking about technical tasks such as mixing. But what about the composition side? AI could shake things up here too. Â
An early attempt to apply machine learning in this field was Magenta Studio, a project from Googleâs Magenta research lab that was made available as a suite of Max For Live tools in 2019. These tools offer a range of takes on MIDI note generation: creating a new melody or rhythm from scratch; completing a melody based on notes given; âmorphingâ between two melodic clips. Trained on âmillionsâ of melodies and rhythms, these models offer a more sophisticated - and, perhaps, more musical - output than traditional generative tools.
AI-powered MIDI note generation has been taken further by companies like Orb Plugins, who have packaged the feature into a set of conventional soft synths â similar to Mixed In Key's Captain plug-ins. Drum sequencers, meanwhile, have begun to incorporate the technology to offer users rhythmic inspiration.
Why the early interest in MIDI? MIDI notation is very streamlined data compared to audioâs 44,000 samples per second, meaning models can be simpler and run lighter. When the technology was in its infancy, MIDI was an obvious place to start.Â
Of course, MIDIâs compactness comes with limitations. Pitches and rhythms are only part of musicâs picture. Addressing the preference for MIDI among machine learning/music hackers a few years ago, the Dadabots wrote: âMIDI is only 2% of what there is to love about music. You canât have Merzbow as MIDI. Nor the atmosphere of a black metal record. You canât have the timbre of Jimi Hendrixâs guitar, nor Coltraneâs sax, nor MC Ride. Pure MIDI is ersatz.â
As AI technology gets more sophisticated and processor power increases, tools are emerging that allow musicians to work directly with raw audio. So are MIDI-based AI tools already a thing of the past?Â
Probably not. Most modern musicians rely on MIDI and other âsymbolicâ music languages. Electronic producers punch rhythms into a sequencer, draw notes in the piano roll, and draw on techniques grounded in music theory traditions (such as keys and modes). AI can offer a lot here. Besides generating ideas, we could use MIDI-based AI tools to accurately transcribe audio into notation, and to perform complex transformations of MIDI data. (For instance, transforming rhythms or melodies from one style or genre into another).
In a talk arguing for the continued importance of âsymbolic music generation,â Julian Lenz of AI music company Qosmo pointed out that raw audio models arenât yet good at grasping the basics of music theory. For example, Googleâs MusicLM, a recent general music model trained on hundreds of thousands of audio clips, has trouble distinguishing between major and minor keys. Lenz concluded by demonstrating a new Qosmo plugin that takes a simple tapped rhythm and turns it into a sophisticated, full-kit drum performance. While raw audio AI tools remain somewhat janky, MIDI-based tools may offer quicker routes to inspiration.Â
Such tools pose tricky questions about the attribution of creativity. If an AI-based plug-in generates a melody for you, should you be considered the âcomposerâ of that melody? What if you generated the melody using an AI model trained on songs by the Beatles? Is the melody yours, the AIâs, or should the Beatles get the credit?
These questions apply to many forms of AI music-making, and weâll return to them in Part 2. For now itâs sufficient to say that, when it comes to MIDI-based melody and rhythm generation, the waters of attribution have been muddied for a long time. Modern electronic composers often use note randomizers, sophisticated arpeggiators, Euclidean rhythm generators, and so on. The generated material is considered a starting point, to be sifted, edited, and arranged according to the music-makerâs creative vision. AI tools may give us more compelling results straight out the gate. But a human subjectivity will still need to decide how the generated results fit into their creative vision.
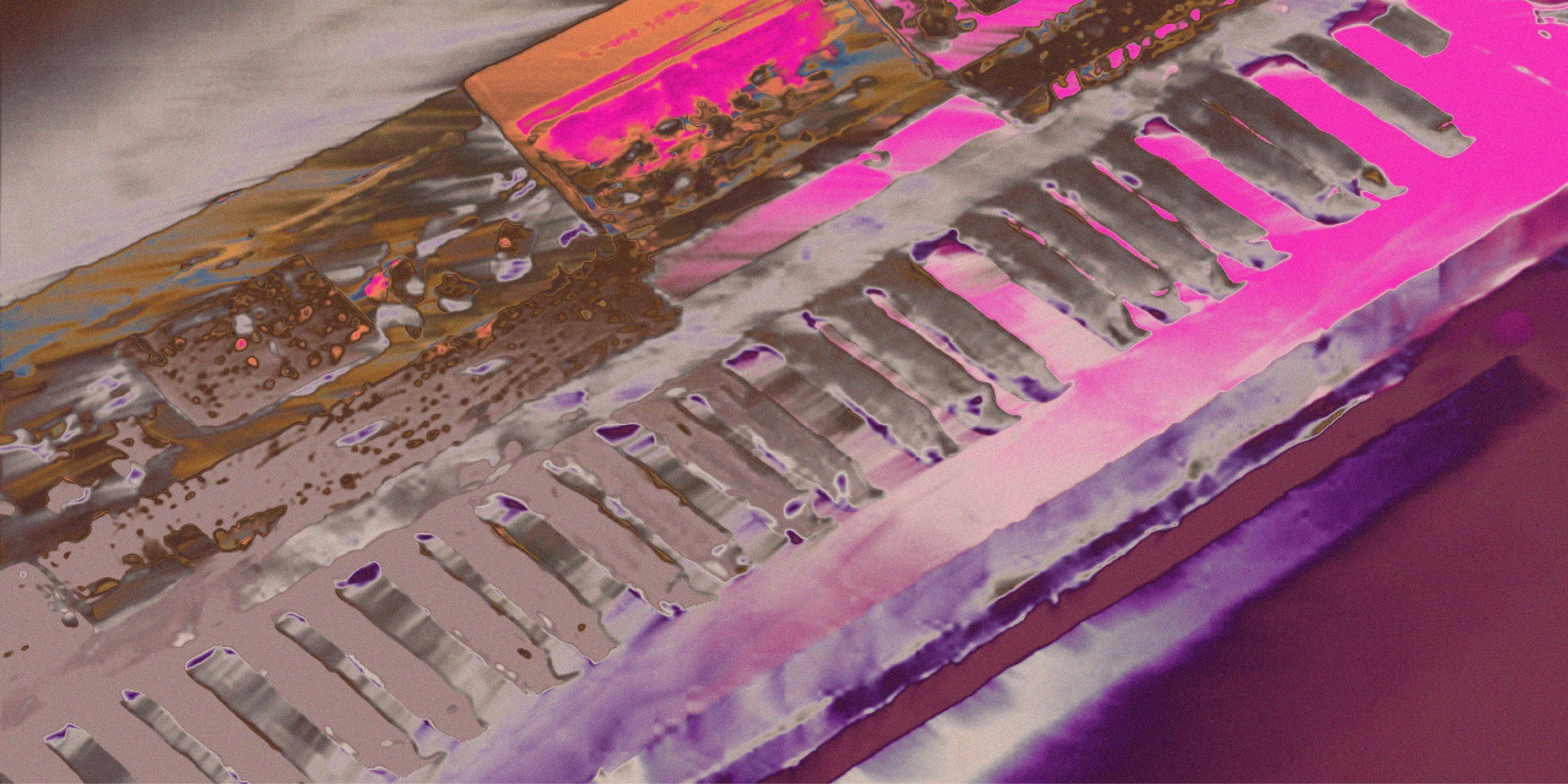
Timbre transfer: Exploring new sounds
When we think of a radical new technology like AI, we might imagine wild new sounds and textures. MIDI is never going to get us there. For this, we need to turn to the audio realm.Â
In the emerging field of âneural synthesis,â one of the dominant technologies is timbral transfer. Put simply, timbral transfer takes an audio input and makes it sound like something else. A voice becomes a violin; a creaking door becomes an Amen break.Â
How does this work? Timbre transfer models, such as IRCAMâs RAVE (âRealtime Audio Variational autoEncoderâ), feature two neural networks working in tandem. One encodes the audio it receives, capturing it according to certain parameters (like loudness or pitch). Using this recorded data, the other neural net then tries to reconstruct (or decode) the input.Â
The sounds that an autoencoder spits out depend on the audio itâs been trained on. If youâve trained it on recordings of a flute, then the decoder will output flute-like sounds. This is where the âtimbre transferâ part comes in. If you feed your flute-trained encoder a human voice, it will still output flute sounds. The result is a strange amalgam: the contours of the voice with the timbre of a flute.
Timbre transfer is already available in a number of plug-ins, though none have yet been presented to the mass market. Perhaps the most accessible is Qosmoâs Neutone, a free-to-download plug-in that allows you to try out a number of neural synthesis techniques in your DAW. This includes RAVE and another timbre transfer method called DDSP (Differentiable Digital Signal Processing). DDSP is a kind of hybrid of the encoder technology and the DSP found in conventional synthesis. Itâs easier to train and can give better-sounding outputs - providing the input audio is monophonic.Â
Timbre transfer technology has been making its way into released music for some years. In an early example, the track âGodmotherâ from Holly Herndonâs album PROTO, a percussive track by the producer Jlin is fed through a timbre transfer model trained on the human voice. The result is an uncanny beatboxed rendition, full of strange details and grainy artifacts.
âGodmotherâ has an exploratory quality, as if it is feeling out a new sonic landscape. This is a common quality to music made using timbral transfer. On A Model Within, the producer Scott Young presents five experimental compositions with just such a quality. Each explores a different preset model found in Neutone, capturing the unfamiliar interaction between human and machine.Â
Even before heâd encountered AI tools, a busy life made Young interested in generative composition approaches. When he started out making music, the producer recalls, âI spent a month making a tune. It was quite romantic. But my life in Hong Kong couldn't allow me to do that too much. And so I slowly attuned to Reaktor generators, to making sequences and stitching them together.â
Last year, the musician Eames suggested that he could speed things up further with generative AI. Young began exploring and came across RAVE, but struggled to get it to work, in spite of his background in software engineering. Then he discovered Neutone. âThe preset models were so impressive that I eagerly began creating tunes with them. The results were mind-blowing. The outputâs really lifelike.â
A typical fear surrounding AI tools is that they might remove creativity from music-making. Youngâs experience with timbre transfer was the opposite. Timbre transfer models are - for now at least - temperamental. The sound quality is erratic, and they respond to inputs in unpredictable ways. For Young, this unpredictability offered a route out of tired music-making habits. âThere's much more emphasis on serendipity in the making [process], because you can't always predict the output based on what you play.â
Once the material was generated, he still had to stitch it into an engaging composition - a process he likened to the editing together of live jazz recordings in an earlier era. âWhen using this generative approach, the key as a human creator is to know where to trim and connect the pieces into something meaningful that resonates with us.â
In the EPâs uncanniest track, âCrytrumpet,â Young feeds a recording of his crying baby daughter through a model trained on a trumpet. Moments like this neatly capture the sheer strangeness of AI technology. But timbral transfer is far from the only potential application of AI in plug-ins.
In March, Steinmetz co-organized the Neural Audio Plugin Competition alongside Andrew Fyfe of Qosmo and the Audio Programmer platform. The competition aimed to stimulate innovation by offering cash prizes for the most impressive entries. âAs far as making neural networks inside plugins, it really hadn't been established yet,â says Steinmetz. âWe need a way to encourage more people to work in this space, because I know there's stuff here to be done that's going to be really impactful.â
Of the 18 entries, some offered neural takes on conventional effects such as compression, and others proposed generative MIDI-based tools. Then there were the more surprising ideas. Vroom, a sound design tool, allows you to generate single sounds using text prompts. HARD is a novel âaudio remixer,â enabling you to crossfade between the harmonic and rhythmic parts of two tracks independently. Everyone was required to open source their code, and Steinmetz hopes future plug-in designers will build on this work. He sees the start of a âmovement of people interested in this topic.â
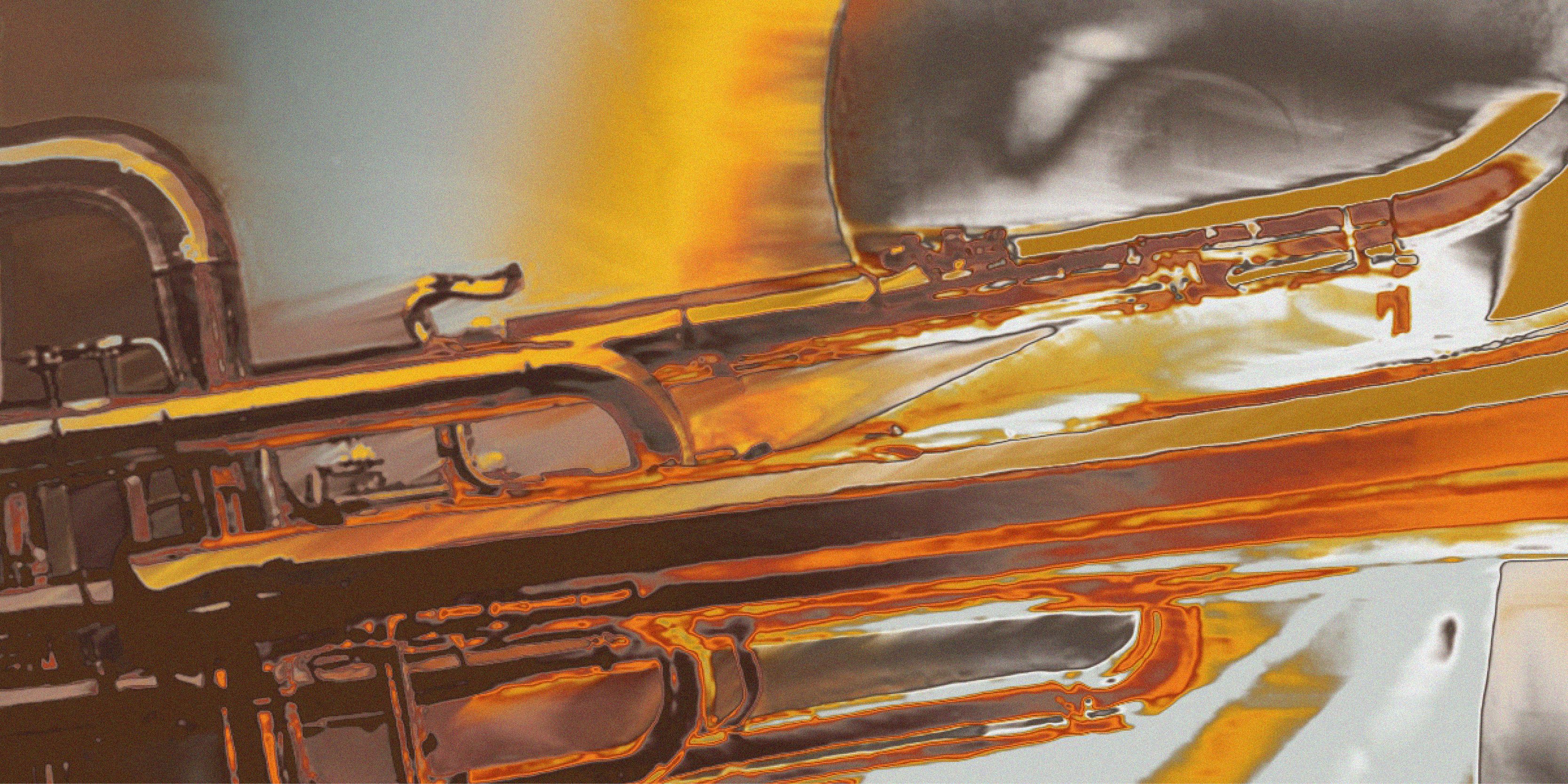
Analog modeling
So, AI can do new sounds. But it can also do old ones - perhaps better than we could before. Analog modeling is a cornerstone of the plug-in industry. According to some, AI could be its future. Plug-ins like Baby Audioâs TAIP (emulating âa 1971 European tape machineâ) and Tone Empireâs Neural Q (âa well-known vintage German equalizerâ) use neural network-based methods in place of traditional modeling techniques.
Baby Audio explain how this works on their website:Â
âWhere a normal DSP emulation would entail âguesstimatingâ the effect of various analog components and their mutual dependencies, we can use AI / neural networks to accurately decipher the sonic characteristics that make a tape machine sound and behave in the way it does. This happens by feeding an algorithm various training data of dry vs. processed audio and teaching it to identify the exact characteristics that make up the difference. Once these differences have been learned by the AI, we can apply them to new audio.â
Why use AI instead of traditional modeling methods? One reason is better results. Tone Empire claims that traditional circuit modeling âcan never produce as authentic an analog emulationâ as AI-based approaches.
Another is speed. Analog modeling using neural processing could potentially save a lot of time and money for plug-in companies. This means we might be looking at a proliferation of low-cost, high-quality analog models - no bad thing for producers who enjoy playing with new toys.
More radically, it means that modeling can be placed in the hands of music-makers themselves. This is already happening in the guitar world, via companies like TONEX and Neural DSP. Neural DSPâs Quad Cortex floor modeling unit comes with an AI-powered Neural Capture feature that allows guitarists to model their own amps and pedals. Itâs simple: the Quad Cortex sends a test tone through the target unit and, based on the output audio, creates a high quality model in moments.Â
This presents exciting possibilities. Many of us have that one broken old pedal or piece of rack gear whose idiosyncratic sound we love. What if you could model it for further use in-the-box - and share the model with friends? Until now, modeling has mostly been the domain of technical specialists. Itâs exciting to think what musicians might do with it.
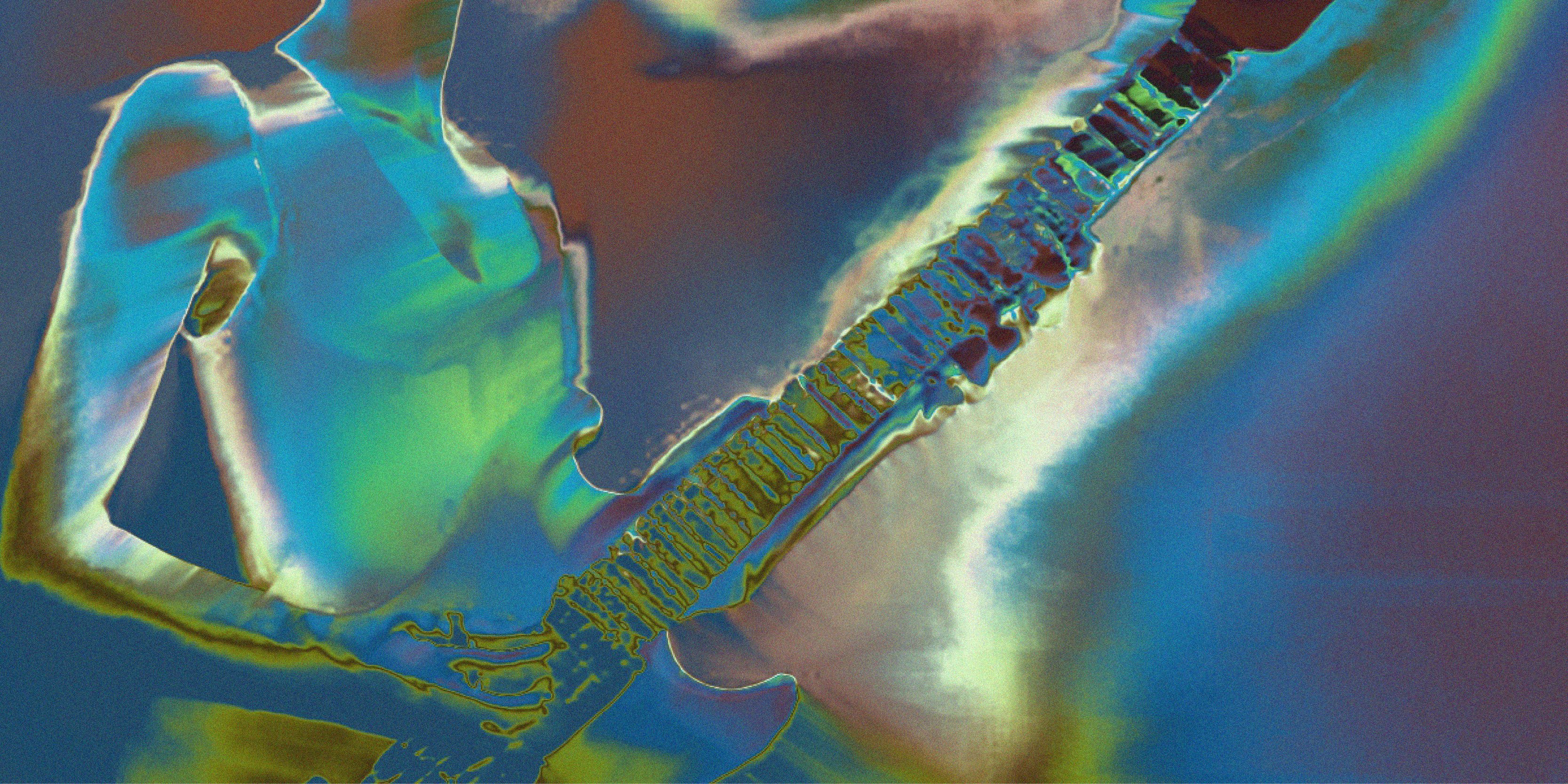
Democratizing music tech
This theme - of bringing previously specialized technical tasks into the hands of musicians - recurs when exploring AI music-making tools. For Steinmetz, analog modeling is just one application of deep learning technology, and not the most exciting. He invites us to imagine a tool like Midjourney or Stable Diffusion, but instead of producing images on command, it generates new audio effects.Â
â[This] enables anyone to create an effect, because you don't need to be a programmer to do it. I can search a generative space - just how I might search Stable Diffusion - for tones or effects. I could discover some new effect and then share that with my friends, or use it for my own production. It opens up a lot more possibilities for creativity."
We looked earlier at how certain production tasks may be automated by AI, freeing up musicians to focus their creativity in other areas. One such area might be the production tools theyâre using. AI technology could enable everyone to have their own custom music-making toolbox. Perhaps making this toolbox as creative and unique as possible will be important in the way that EQing or compression is today.
Steinmetz envisions âthe growth of a breed of programmer/musician/audio engineer, people that are both into the tech and the music side.â These people will either find creative ways to âbreakâ the AI models available, or âbuild their own new models to get some sort of new sound specifically for their music practice.â He sees this as the latest iteration of a longstanding relationship between artists and their tools. âWhenever a [new] synthesizer is on the scene, there's always some musicians coming up with ideas to tinker with it and make it their own.â
Dryhurst also sees a future in artists building their own custom models, just as he and Herndon have done for PROTO and other projects. âI feel that is closer to how many producers will want to use models going forward, building their own ârigâ so to speak, that produces idiosyncratic results. I think that over time, we might also begin to see models themselves as a new medium of expression to be shared and experienced. I think that is where it gets very exciting and novel; it may transpire that interacting with an artist model is as common as interacting with an album or another traditional format. We have barely scratched the surface on the possibilities there yet.â
Read Part 2 of this article.
Text: Angus Finlayson
Images: Veronika Marxer
Have you tried making music with AI tools? Share your results and experience with the Loop Community on Discord. If youâre not already a member, sign up to get started.